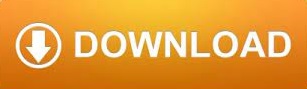
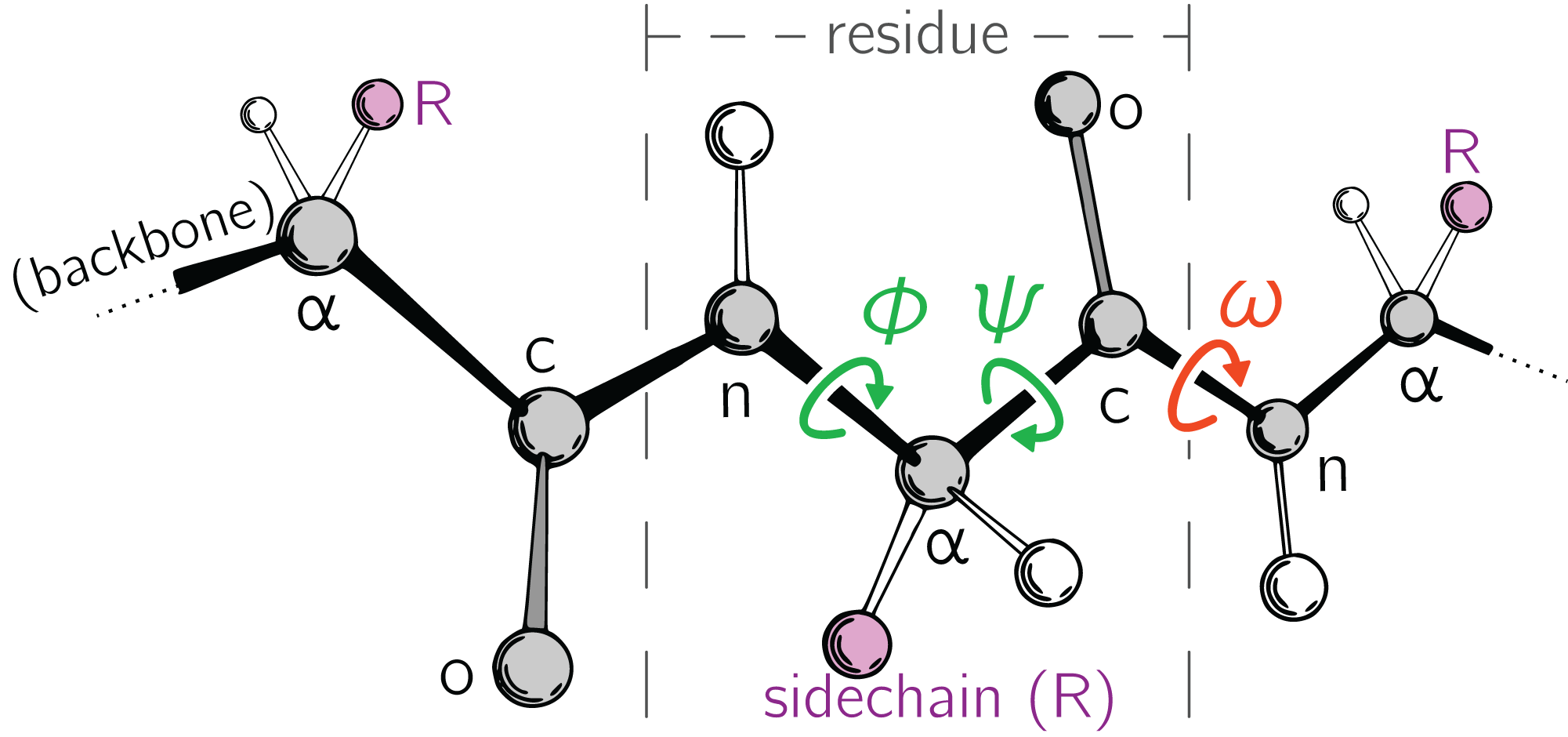
The BBQ package is available for downloading from our website at This webpage also provides a user manual that describes BBQ functions in detail. Obtained results provide a comprehensive benchmark of existing tools and evaluate their applicability to a large scale modeling using a reduced representation of protein conformational space. BBQ (Backbone Building from Quadrilaterals) program has been extensively tested both on native structures as well as on near-native decoy models and compared with the different available existing methods. Most methods that predict the local structure of a protein from its sequence first rely on some classification and only then. For each group, a single protein record was generated with each point in the amino acid sequence annotated with the, backbone dihedral angles averaged over all the structures in the record. Motivation Local protein structure is usually described via classifying each peptide to a unique class from a set of pre-defined structures. These classifications may differ in the number of structural classes, the length of peptides, or class attribution criteria. The details and careful design of the present approach are new and lead to the algorithm that outperforms all commonly used earlier applications. Motivation Local protein structure is usually described via classifying each peptide to a unique class from a set of pre-defined structures. The method employed in this work follows the main idea of some earlier approaches to the problem. The peptide chain is known as the backbone, and the 'R' groups are known as side chains. SCUBA-D has been developed by gradually adding new components to a. Here we report SCUBA-D, a method using denoising diffusion with priors of non-zero means to transform a low quality initial backbone into a high quality backbone. Reconstruction of the main chain atomic coordinates from the alpha carbon trace is a common task in protein modeling, including de novo structure prediction, comparative modeling, and processing experimental data. In de novo deisgn of protein backbones with deep generative methods, the designability or physical plausibility of the generated backbones needs to be emphasized. In this contribution, we present an algorithm for protein backbone reconstruction that comprises very high computational efficiency with high accuracy. Source: Journal of Computational Chemistry, 28:1593–7, 2007 Abstract
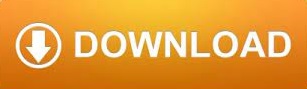